Interview: Professor Gordon Cheng on new learning strategies for robots
“Having a purpose is extremely powerful”
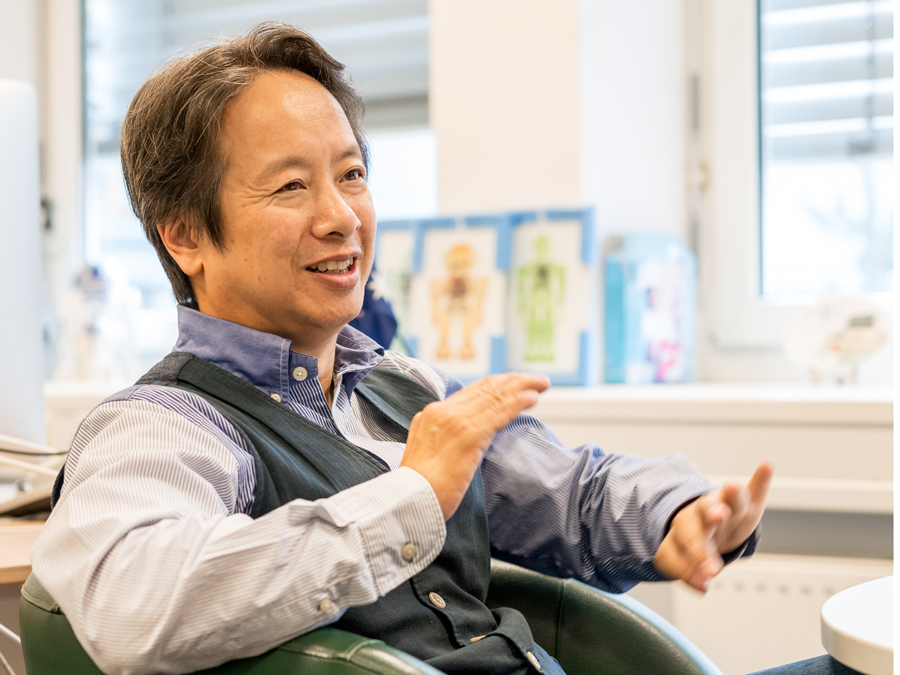
Could you explain what you mean by “purposive learning”?
Humans and animals have three basic strategies of imitating others and, by doing so, learning. The first approach is appearance-based. This basically means exactly mimicking movements as they appear to us. The second strategy is action-based – focusing on learning what action to take. The third approach is purpose-based: Which means understanding the purpose of an action. This doesn’t only make it easy to learn from others, it also makes us more flexible in performing these actions.
Can you give an example?
When doing the dishes, for example, our purpose is to get those plates and cups clean. We can adapt to different kitchen designs, cleaning equipment and items to be washed because we know why we do what we are doing. Having a purpose is extremely powerful.
How does this apply to robots?
If you want to teach a robot a task, it makes no sense to take the appearance-based approach and have it exactly imitate a human. For example, most robots have bodies very different from humans. Direct mapping, i.e. exactly copying a particular human's movement while doing the dishes will probably only lead to a broken plate. If you take the action-based approach and carefully program a robot's movements, it will be able to effectively wash the plate. However, when presented with a cup or just a plate with a different size, it will fail.
Which would be the benefits of a purpose-based approach?
Ideally, we want to explain a robot that we want our dishes cleaned and have it clean them without any further need for programming. The general idea here is to teach knowledge to robots and enable them to reason about that knowledge. In our dishwashing scenario, the robot has a general idea about what “dishes” are and it knows, what “clean” means, as well as the actions it needs to perform to clean the dishes. This robot knows that a cup and a plate both are objects that can be washed and it can adapt its cleaning strategies accordingly.
How do you teach knowledge to a robot?
One of the tools we use to represent knowledge is a network of ontologies. The world as humans perceive it can be expressed in ontologies, in relations between an object and your actions. For each object, you can reason about which actions are possible. This begins with our body: We know which actions our arm can perform, our right hand, and each digit of our fingers. It’s also true for other objects, such as, a sponge: You will very likely not use it to cut a sausage. Instead, you have formed a mental ontology that tells you that the possible actions for a sponge would be soaking it in water and rubbing it over something. Shared ontologies make it very easy to explain actions to others.
But how do you explain this to a machine? Do you sit down and compose massive databases of relations?
One way of doing this is to look at a large number of humans performing a task and analyzing their actions. In our lab, we actually use washing dishes as a way of creating knowledge: We created a kitchen sink in a virtual reality environment and have lots of people with different bodies and washing styles clean virtual dishes. With special software, it's possible to break down their actions and create meaningful data.
Do you have to do this all over for each new task?
No. Once you create a relationship database, you can use it for other tasks. Researchers have been creating such common sense databases for a while now. There often already is an existing database to provide you with the basics. Then you can build on top of that.
Are there other uses than just teaching everyday tasks to robots?
The ability to take human actions apart and teach machines to make sense of them offers many new possibilities. One thing that could be interesting for future research is human-robot collaboration in an industrial setting: Once a machine understands what you are doing and is able to conclude what you will – or should - be doing next, it can assist you much more effectively.
Publication:
G. Cheng, K. Ramirez-Amaro, M. Beetz, Y. Kuniyoshi: "Purposive Learning: Robot Reasoning about the Meanings of Human Activities", Science Robotics, 2019. DOI: 10.1126/scirobotics.aav1530
More information:
Gordon Cheng holds the Chair of Cognitive Systems at TUM. He is the coordinator of the Center of Competence NeuroEngineering and one of the key researchers at the Munich School of Robotics and Machine Intelligence (MSRM), a research center at TUM dedicated to interdisciplinary research into the future of health, work and mobility.
Contact:
Prof. Dr. Gordon Cheng
Technical University of Munich
Chair of Cognitive Systems
Phone: +49 (89) 289-25765
borngesser
@tum.de
High-resolution images:
Technical University of Munich
Corporate Communications Center
- Paul Hellmich
- paul.hellmich @tum.de
- presse @tum.de
- Teamwebsite