Macro-assemblies for medical applications and energy production
With machine learning to new supramolecular materials
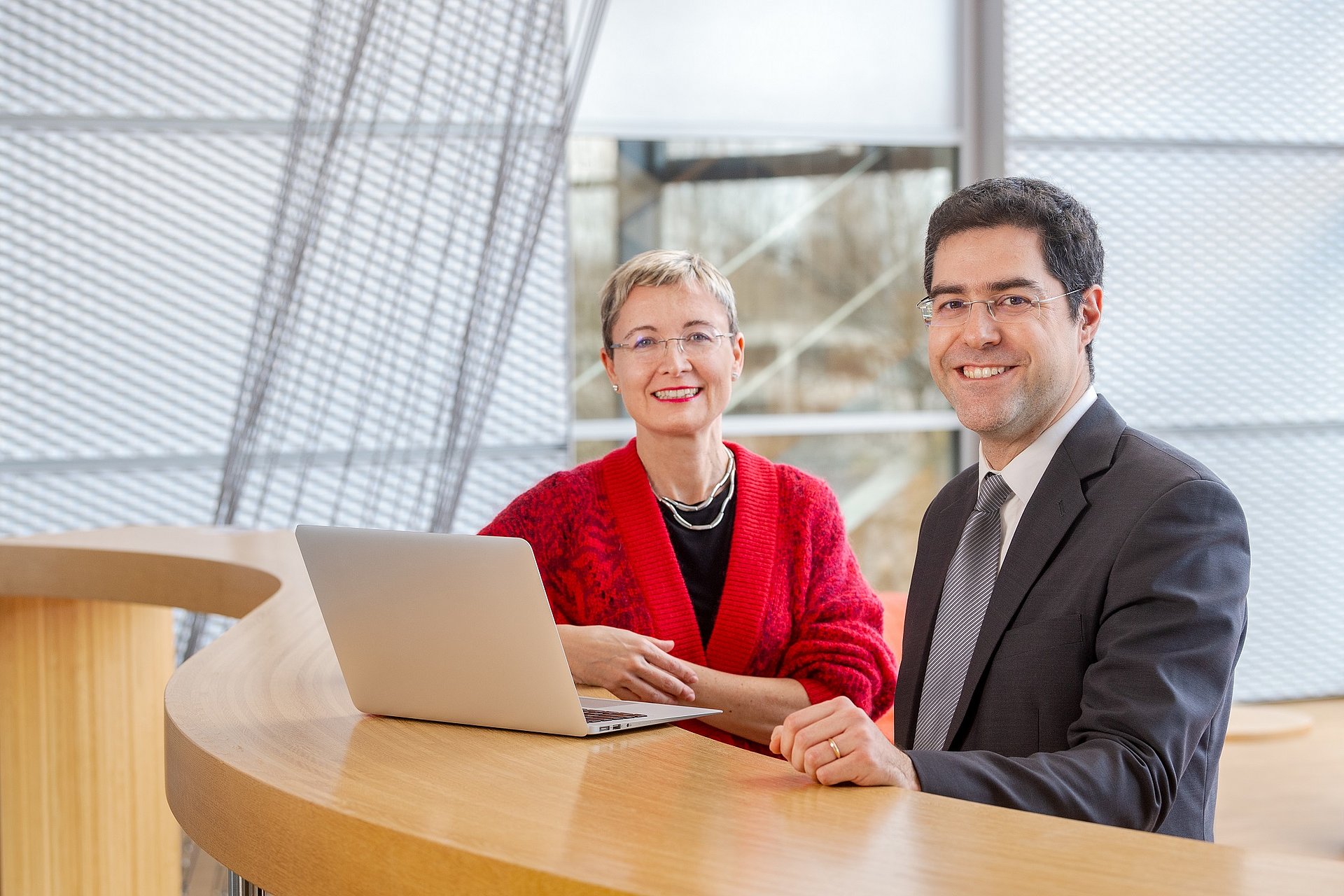
A team of scientists at the TUM Innovation Network ARTEMIS (Artificial Intelligence Powered Multifunctional Material Design), named after the Greek goddess of hunting, are researching supramolecular materials. They are investigating their use in medicine and energy production supported by machine learning. “The idea of ARTEMIS is to teach machines to find the main determinants for material design,” says Alessio Gagliardi, one of the two coordinators of the network and Professor for Simulation of Nanosystems for Energy Conversion at the Technical University of Munich (TUM).
The researchers want to show the broad range of applications of supramolecular materials. That is why they have chosen to test their techniques on medical applications, such as implant coverings, as well as electro-catalysis for water splitting in hydrogen production.
One of the main problems with medical implants, for example, is the growth of bacteria, which can coat the surface of a device and cause infections. New covering materials that keep bacteria from developing by actively releasing drugs or by forming a repellant surface could be the solution. The properties of supramolecular systems make them powerful tools to fulfill such functions and constitute an ideal platform to discover novel “smart” multicomponent coatings.
Combining Supramolecular Materials for New Applications
Supramolecular materials are a broad and diverse class of materials. They consist of different molecular building blocks that can be combined in many ways. These macro-assemblies can consist of a variety of elements like carbon or metals. Some of them can form cages that trap other chemical compounds. The singular building blocks are held together via so-called non-covalent interactions like hydrogen-bonding and hydrophobic or electrostatic interactions. Compared to covalent bonds they are much more diverse and potentially reversible.
“Because of the great diversity of supramolecular materials, it is possible to generate an incredible number of compounds with different characteristics, making them fantastic tools in many applications,” says Angela Casini, co-coordinator of the network and Professor of Medicinal and Bioinorganic Chemistry. “But because of the large variety of possible combinations, finding the best out of billions is a bit like looking for a needle in the haystack,” she adds.
Making Machines Smarter
This is where machine learning comes in. The team of scientists has a few different plans to integrate Artificial Intelligence into materials science. “Our main goal is to teach machines to predict the best combination of molecules for a particular set of future applications. We therefore need to learn how much data and what type of data about a supramolecular material we have to provide to the machine in order to predict the most appropriate combinations,” says Gagliardi. Another way to use the potential of machine learning is to accelerate directly atomistic simulations of molecular properties.
In hydrogen production, one of the research fields of ARTEMIS, fuel cells are disposed of, which mainly use platinum as a catalyst. The problem is that platinum is very expensive, which means high performance must be based on a low platinum load. One can achieve this by nanostructuring platinum into nanoparticles with specific shapes and sizes.
But there is to date no way of synthesizing specific nanoparticles in large amounts. “If we could create structures of metal-organic frameworks (MOFs) that can trap the platinum-precursor very effectively, like nano-cages, we could produce the correct nanoparticle with high precision and decrease the amount of platinum needed. Cars with hydrogen power would then be less expensive,” explains Gagliardi. The team is searching for supramolecular compounds to develop more effective MOFs with the help of computational calculations.
Connecting the University with the ARTEMIS Innovation Network
In the TUM Innovation Network ARTEMIS, researchers from five university departments—Chemistry, Electrical and Computer Engineering, Informatics, Engineering, and Physics—work together. They cooperate with the TUM Catalysis Research Center and the Munich Data Science Institute. “In the beginning, we had to struggle with communication problems between people from the different disciplines. We had to learn to understand each other’s scientific languages—chemists use different terminology to engineers. But this exchange opened our minds and paved the way to new ideas,” says Casini.
Besides initial problems, the cooperation across disciplines has yielded the first scientific success: The team applied machine learning algorithms to distinguish the surfaces of different variants of biofilms and plant leaves. By identifying critical surface features they were able to correctly classify them according to their wettability.
About TUM Innovation Networks
The new TUM Innovation Networks bring together transdisciplinary teams to unleash their collective creativity and scientific expertise to form high-potential innovation fields at the interface of traditional disciplines. Each project, like ARTEMIS, runs for four years and is supported by the university with an overall budget of approximately €3 million. Innovation Network teams consist of as many as ten doctoral candidates and postdocs in addition to between 7 and 10 Principal Investigators. Every year, one or two new networks are selected in a three-step evaluation process. The TUM Innovation Networks are central to TUM’s Agenda 2030 and are supported by the Federal Ministry of Education and Research (BMBF) and the Free State of Bavaria under the Excellence Strategy of the Federal Government and the Länder.
The article was already published on the website "Research in Bavaria" of the Bavarian State Ministry of Science and Arts.
Link zu the article about ARTEMIS on the website "Research in Bavaria"
Technical University of Munich
Corporate Communications Center
- Dr. Vera Spaett
- vera.spaett @tum.de
- presse @tum.de
- Teamwebsite